Apple Intelligence and the Adoption Chasm
When I heard about Apple’s plans to roll out AI features in MacOS, it brought me back to the mid–’90s to the moment when companies started putting their web addresses in television commercials. I remember seeing those URLs on TV because, even at the time, I knew I was witnessing the web going mainstream. I'm not ready to predict how we'll look back on the beginning of Apple Intelligence, but I did give it a try on my Mac and it sparked a few thoughts about where things stand for broader adoption of AI technology.
But before we dig into Apple Intelligence, let's take a brief detour through one area of communication theory.
In his 1962 book Diffusion of Innovations, communication theorist Everett Rogers suggested that new technologies spread through culture in phases that map onto a normal distribution:
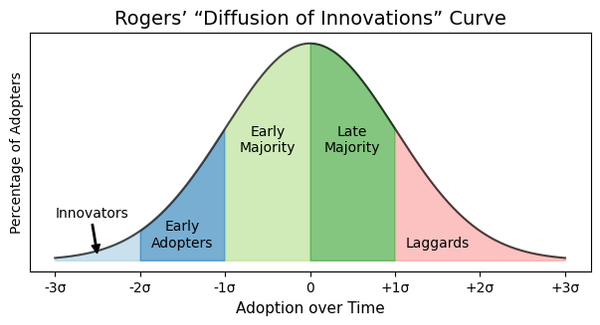
According to Rogers, in the earliest days of a new technology, only a tiny sliver of "innovators" are willing to use it. (Most people haven't even heard of it at this stage.) As the technology develops, it attracts the attention of a larger group of tech-adventurous folks called “early adopters.” Some of these early adopters will discover valuable applications that attract the attention of a still-larger group of people. At some point—namely at 16% adoption if you accept Rogers' idea that this all fits a normal distribution—everyday non-technical people (the “early majority”) start adopting it. At this point the technology has gone mainstream.
This is all very beautiful on a conceptual level, with the symmetry and gracefulness of the bell curve implying an orderly coherence to the adoption of a new technology. (It also subtly implies that diffusion is inevitable, and that those who hesitate—the “laggards”—are morally suspect. This way of thinking, when it takes a more extreme form, is what L.M. Sacasas calls the Borg Complex.)
But there is an interesting wrinkle to this beautiful curve. In 1989, a group of business consultants started discussing an observation they'd made about the behavior of certain real-world technologies with respect to Rogers' curve. They had noticed that some technologies, even those that seemed to be on the fast track to broad adoption, get stuck when they reach the transition from early adopters to early majority. Sometimes, technologies that are a hit with early adopters just never gain traction in the broader population. Other times the technology does eventually make the jump, but only on the second or third try (and sometimes under the auspices of a company other than the one that developed it).
They called this point of discontinuity the “marketing chasm.“
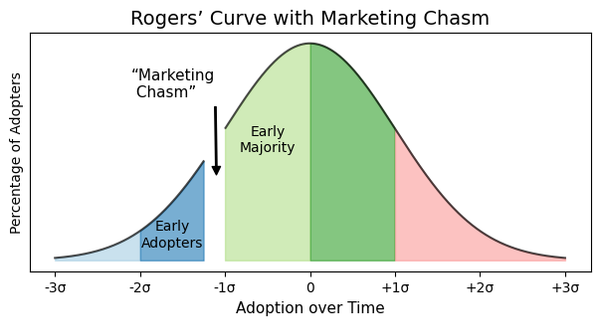
It's called the “marketing” chasm because it derives from a basic principle of communication: understanding your audience. The idea is that there is often a qualitative difference between what motivates early adopters and what motivates the early majority. Early adopters are drawn to new technologies because they are new. They enjoy trying new things whether they work well or not. The early majority, on the other hand, is not interested in novelty for its own sake. They want tools that work. They're willing to learn a few new things to get there, but they don't have nearly the patience that early adopters do for half-baked solutions.
This is the danger of the chasm. A technology could have all the promise in the world, and legions of excited early adopters who want to help it spread. But if those early adopters—or, more pointedly, a company that has developed a new technology and who wants to sell it to a broader market—fail to present it in a way that resonates with the broader audience, progress will halt.
It seems to me that the use of LLMs for general computing workflows1 is somewhere in the vicinity of the marketing chasm right now. While I believe that LLMs have so much latent power that there's no question whether they'll make the leap to adoption by the majority market, I do think there are at least three major open questions:
- How long will it take before just about everyone is leveraging LLMs for basic personal computing workflows?
- What will this look like once it happens?
- Who will figure out how to profit from it?
This brings us back to Apple, a company whose history we can understand better with the marketing chasm in our conceptual toolbelt. In fact, you might say that Apple has built its success on its ability to traverse the chasm and be the first to tap into lucrative mass markets for new technologies. The graphical user interface, the MP3 player, the smartphone… Apple did not originate any of these technologies. But in each case they were the first to market a product that succeeded with a mass audience.
So if Apple is the expert in introducing a new technology to a mass audience, what does Apple Intelligence show us about the future of LLMs in the majority market?2
The first thing I noticed is that using the new AI features is still very much an opt-in experience. Just updating to MacOS 15.1 doesn't enable them; you also have to flip a master switch in the system settings. I hadn't expected this given how much attention Apple has given it in their advertising. This is a sign of caution on Apple's part: they're limiting the exposure of the technology to people who specifically seek it out. In this respect, they're just dipping their toes in the early majority audience rather than diving in.
I also noticed that the AI features are quite modest in their presentation. Unlike AI features in other software I've seen, Apple Intelligence is not flashy; it doesn't particularly draw attention to the fact that it's AI. In other words, Apple is not aiming for whiz-bang with these features. They're aiming to improve the user's ability to accomplish certain tasks through targeted application of some new capabilities. This kind of move makes sense in the transition from early adopters to early majority.
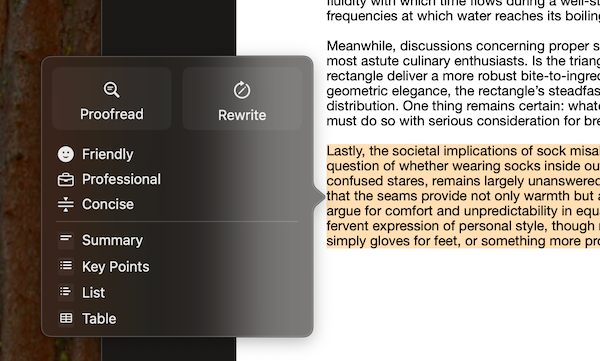
The last observation I'll make is that the features are tightly focused. That's the positive way to say it; “limited” would also work. Early adopters who are used to interacting with LLMs via prompts will notice how much less freedom Apple Intelligence gives them. Instead of the wide-open field of a free-text prompt, you get a dialog box with a handful of pre-defined buttons such as “proofread,” “make friendly,” and “summarize.” This too makes sense in light of the marketing chasm: what early adopters see as powerful versatility, the early majority sees as bewildering complexity. But this isn't just a user-facing benefit. By reducing the scope of what its built-in LLM can do, Apple may be able to avoid the embarrassments that have followed the launch of branded LLMs (e.g. Gemini) that give users the freedom of motion to construct full prompts.
What remains to be seen is whether this constricted user experience is just a transitional step, or if it will remain the shape of mass LLM deployment moving forward. This will be worth watching, because in the long run the most consequential interface to LLMs will not be the chatbot (where the fact that one is using an LLM is front-and-center). Rather, LLMs will have the greatest impact through the as-yet-undetermined ways in which they are woven into what we think of as everyday computing.
Footnotes
1: I'm highlighting the use of LLMs in "general computing workflows" in contrast to the chatbot, despite the latter being by far the most widely-deployed mode for LLMs.
2: I know that Apple Intelligence is about more than LLMs, but LLMs are a substantial part of it. They're also what I'm most interested in.